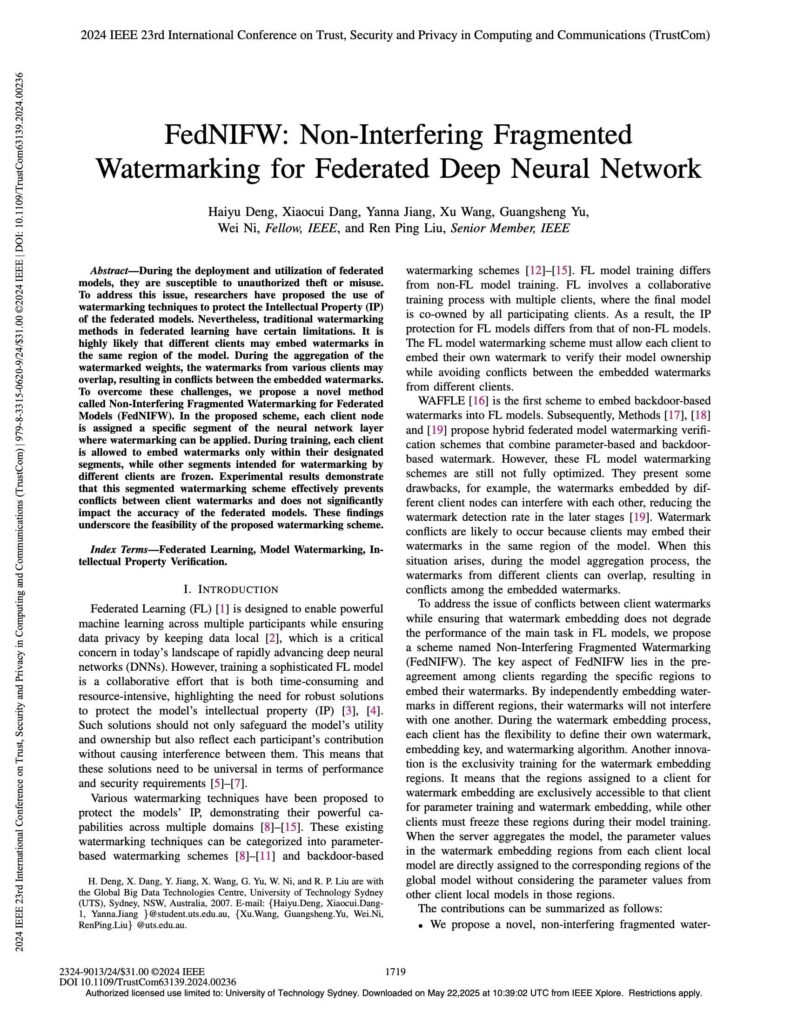
FedNIFW: Non-Interfering Fragmented Watermarking for Federated Deep Neural Network
Haiyu Deng, Xiaocui Dang, Yanna Jiang, Xu Wang, Guangsheng Yu, Wei Ni, Ren Ping Liu
2024 IEEE 23rd International Conference on Trust, Security and Privacy in Computing and Communications (TrustCom)
During the deployment and utilization of federated models, they are susceptible to unauthorized theft or misuse. To address this issue, researchers have proposed the use of watermarking techniques to protect the Intellectual Property (IP) of the federated models. Nevertheless, traditional watermarking methods in federated learning have certain limitations. It is highly likely that different clients may embed watermarks in the same region of the model. During the aggregation of the watermarked weights, the watermarks from various clients may overlap, resulting in conflicts between the embedded watermarks. To overcome these challenges, we propose a novel method called Non-Interfering Fragmented Watermarking for Federated Models (FedNIFW). In the proposed scheme, each client node is assigned a specific segment of the neural network layer where watermarking can be applied. During training, each client is allowed to embed watermarks only within their designated segments, while other segments intended for watermarking by different clients are frozen. Experimental results demonstrate that this segmented watermarking scheme effectively prevents conflicts between client watermarks and does not significantly impact the accuracy of the federated models. These findings underscore the feasibility of the proposed watermarking scheme.