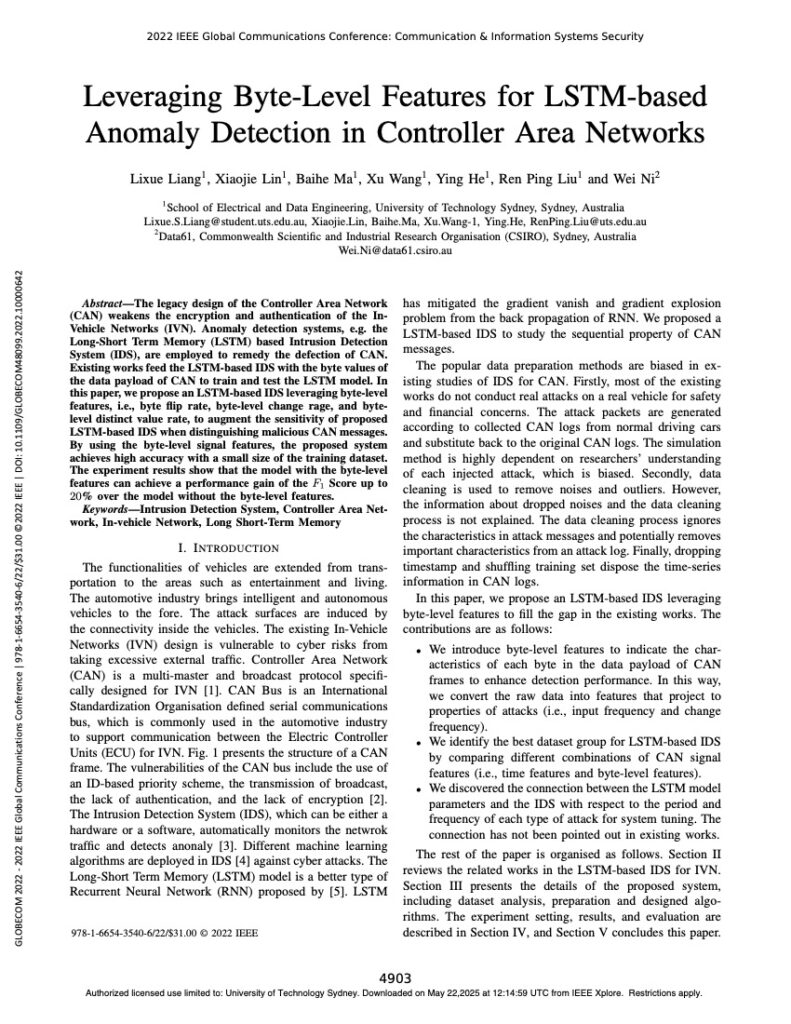
Leveraging Byte-Level Features for LSTM-based Anomaly Detection in Controller Area Networks
Lixue Liang, Xiaojie Lin, Baihe Ma, Xu Wang, Ying He, Ren Ping Liu, Wei Ni
GLOBECOM 2022-2022 IEEE Global Communications Conference
The legacy design of the Controller Area Network (CAN) weakens the encryption and authentication of the In-Vehicle Networks (IVN). Anomaly detection systems, e.g. the Long-Short Term Memory (LSTM) based Intrusion Detection System (IDS), are employed to remedy the defection of CAN. Existing works feed the LSTM-based IDS with the byte values of the data payload of CAN to train and test the LSTM model. In this paper, we propose an LSTM-based IDS leveraging byte-level features, i.e., byte flip rate, byte-level change rage, and byte-level distinct value rate, to augment the sensitivity of proposed LSTM-based IDS when distinguishing malicious CAN messages. By using the byte-level signal features, the proposed system achieves high accuracy with a small size of the training dataset. The experiment results show that the model with the byte-level features can achieve a performance gain of the F1 Score up to 20% over the model without the byte-level features.